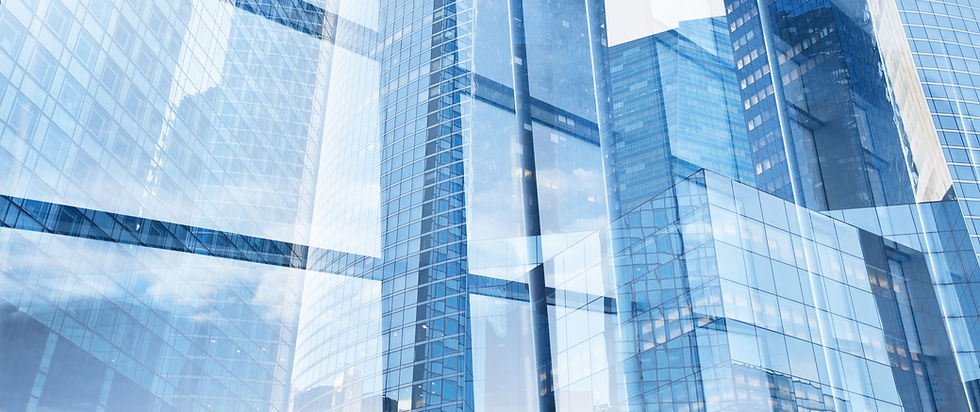
Is the Financial System Fit for Purpose?
Research Project
Deep Learning in a New Keynesian Model of Banking and Money Creation
Scroll Down

Principal Investigator: Dr Mingli Chen
Mingli Chen is Associate Professor of Economics at the Universiy of Warwick. Her research fields are Econometrics, Time Series Econometrics, Financial Econometrics and Industrial Organization. In particular, her papers are on big data (observations and/or variables are large), detection of structural breaks, large network estimation and the study of consumer payment choice.
​
Co-Investigators: Andreas Joseph (Bank of England), Michael Kumhof (Bank of England) and Aruhan Shi (University of Warwick).

Project Summary
This project tries to address one of the criticisms faced by Dynamic Stochastic General Equilibrium (DSGE) models since the Global Financial Crisis 2008, namely the unrealistic implications of the rationality assumption for economic behavior. DSGE models with rational expectations agents are widely used in macroeconomic analysis and are workhorse models for monetary policy analysis at central banks, including the Bank of England. Addressing the criticisms directed at expectations formation is thus highly policy relevant. In this project, we address this through a DSGE model structure where rational expectations are replaced by artificially intelligent (AI) agents that via deep reinforcement learning (DRL).
Specifically, we consider a model in which agents (households) have access to fiat money and nominal government bonds, and seek to maximize their utility, which depends on consumption, real money balances and hours worked, subject to an inter-temporal budget constraint. Agents only observe their actions, realized macroeconomic variables, and the resulting utility, while they have limited knowledge of the structure of the economy. Agents’ expectations affect how the aggregate economy is affected by disturbances. Applying DRL enables a flexible learning process compared with conventional macro learning models. In this setting, an agent can learn appropriate transition functions given a sufficient training history, which naturally allows for path dependency. Moreover, DRL has an important technical advantage – it is able to solve for solutions in an environment with large action and state spaces.
We want to answer several timely questions through this project. First, when facing different combinations of monetary and fiscal policies (active/passive monetary/fiscal policies), what are the differences between learnt equilibria under different learning approaches? Second, what are the solutions of well-known macroeconomic models within a framework of agents that use a form of learning derived from artificial intelligence? Third, under which conditions is the rational equilibrium solution obtained in the long run? Fourth, what are the implications for monetary policy, especially in the presence of large shocks which may drive the system to the zero lower bound or into a liquidity trap?
Other than being policy relevant, this project identifies a promising area of future research which has not yet received much attention, namely the intersection between learning in macroeconomics and in the new field of artificial intelligence. As such, the project is also interdisciplinary and aims at the development of new and practical methods.

Results
Deep Reinforcement Learning in a Monetary Model
Mingli Chen, Andreas Joseph, Michael Kumhof, Xinlei Pan, Rui Shi, and Xuan Zhou | April 21, 2021