Artificial Intelligence in a Monetary Model
- Rebuild Macro
- Apr 22, 2021
- 4 min read
By Mingli Chen (University of Warwick), Andreas Joseph* (Bank of England), Michael Kumhof* (Bank of England), Xinlei Pan (UC Berkeley), Aruhan Shi (University of Warwick), Xuan Zhou (Deakin University)
In our project for Rebuilding Macroeconomics, we use deep reinforcement learning algorithms to solve dynamic stochastic general equilibrium models. Specifically, we populate a monetary model with Artificial Intelligent Agents who learn to solve their dynamic optimization problem by interacting with an environment of which they have no a priori knowledge. This model is well-known from the adaptive learning literature in macroeconomics, and looks at the interaction of monetary and fiscal policy.
Expectation is a central notion in macroeconomics. As a motivating example of the importance of agents’ expectations, below we start with an example of financial stability, which has been at the centre of economic policy discussions since the global financial crisis. Prior to the crisis, finance had rarely been incorporated into mainstream macroeconomic models. Since the crisis, a growing number of models consider the financial sector and its interactions with the rest of the economy in greater detail. Figure 1 shows the annual percentage change of private sector credit in the US. There was a ‘cliff edge’ in credit provision at the onset of the global financial crisis. Standard macro models have a hard time describing such large and sudden moves.
Figure 1: Credit dynamics in the US around the Global Financial Crisis. Source: BIS
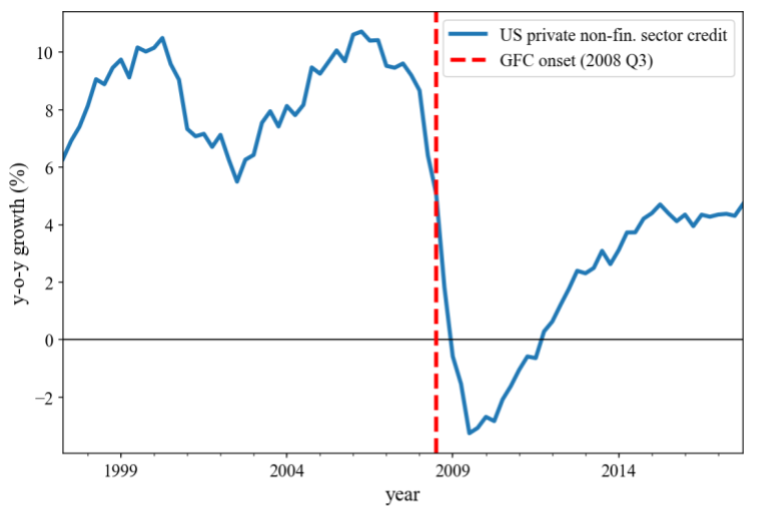
How do such sudden changes in behaviour, like the above contraction in credit, come about? One key channel is abrupt changes in expectations regarding the economic outlook. The standard paradigm of rational expectations under full information, while analytically simple, has come under growing scrutiny due to its unrealistic assumptions. On the other hand, behavioural rules, e.g. the use of simple heuristics to take decisions, can lead to impressive results in modelling micro behaviour but may be judged as arbitrary and as imposing insufficient discipline on a macro model. The number of possible decision rules may be large in problems involving many variables.
We instead propose a middle ground, which may also allow us to uncover decision rules in a rigorous framework.
Learning is making ground in macroeconomics. In learning models, households, consumers, or firms, need time to adjust to new realities and make (understandable) mistakes in their assessment of the future, but adjust their behaviour as new information becomes available.
In this project, we propose to take learning approaches further, by incorporating Artificial Intelligence (AI) into learning and decision processes. In this framework, an AI agent interacts with the environment and chooses an action. But the agent only knows its utility function and observes realized economic variables, while having limited knowledge about the structure of the economy. The agent’s action affects how the current state transitions to the next one, i.e. how the world moves on. In this process, every agent receives rewards or punishments based on their actions, which is then taken into consideration for the future.
Agents’ forward-looking behaviour is based on the revision of past expectations on the basis of observed outcomes, but with limited knowledge of the underlying model. Not only is this framework intuitive and realistic, it also has technical advantages over previous learning approaches in macroeconomics. For example, it introduces a lever which allows us to ‘tune the rationality of agents’. The choice of the desired, or right level, of this tuning is an empirical question and of course may change over time if new information challenges our present understanding. Another advantage is that this approach is able to handle a much larger number of state variables than traditional learning models. We apply our proposed approach to a classical model from the adaptive learning literature in macroeconomics which looks at the interaction of monetary and fiscal policy. We find that, contrary to adaptive learning, the artificially intelligent household can solve the model in all policy regimes.
In our following-up work, we are interested in introducing both private money creation and AI agents into the setting of a New Keynesian Dynamic Stochastic General Equilibrium models to model the dynamics of an economy that copes with large shocks. This will enable us to answer some important, timely, and policy relevant questions: how do AI agents respond to unexpected shocks and how do they differ from currently used rational benchmarks for policy assessment?
What are the implications for credit provision, money creation and financial stability? For instance, given a Lehman Brothers-type event, the reaction of agents will depend on whether and how much they have learned about such an event beforehand. The money creation model by itself can generate extremely large responses to such events, but whether they actually do occur depends on how fast banks and their customers learn and respond. The proposed framework allows us to study this situation in a rigorous framework while incorporating realistic mechanisms for money creation and expectations formation.
*Disclaimer: The views expressed here are those of the authors alone and do not necessarily reflect those of the Bank of England or any of its committees.
Comments